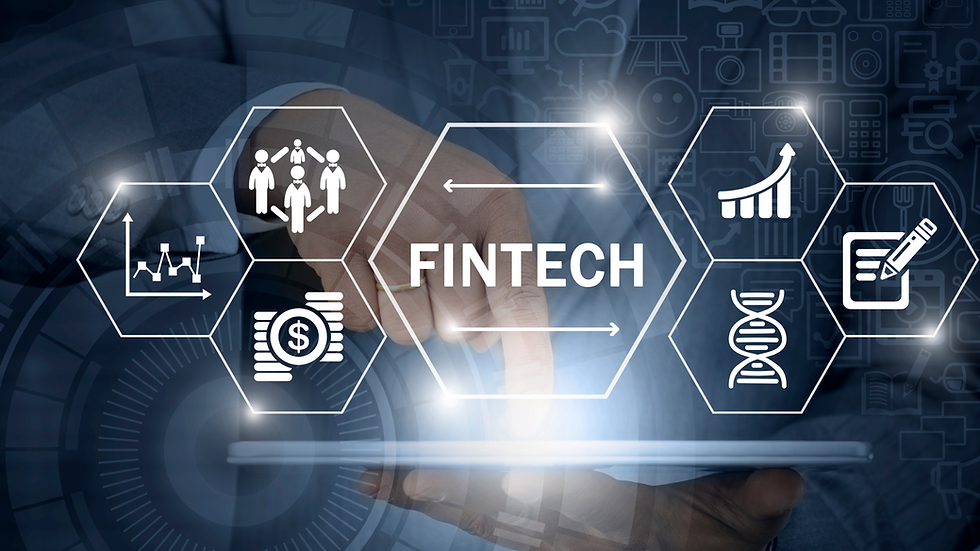
Introduction
The delivery of financial services might be completely changed by the convergence of artificial intelligence (AI) and financial technology (fintech). AI may be applied to financial transactions to boost speed, accuracy, and efficiency while lowering costs.
Fintech's usage of AI has the potential to revolutionize the financial sector by enhancing client experience, lowering fraud, and expediting investment management. Fintech's use of AI, however, also brings with it a number of difficulties, including the need for increasingly sophisticated AI methods to manage complicated financial transactions and worries about data privacy and security. Despite these difficulties, AI in fintech offers prospective advantages that make it a field worth investigating for businesses in the financial sector.
How AI is being used in fintech: Risk assessment and fraud detection
Risk evaluation and fraud detection are two of the major applications of AI in finance. AI can examine enormous volumes of data to find patterns and abnormalities that can point to fraud. In comparison to more conventional techniques, this enables more precise and effective fraud detection.
Machine learning algorithms are one way that AI is being utilized in fintech to detect fraud. In order to find trends that can point to fraudulent conduct, these algorithms can examine data from a variety of sources, including client transaction histories and account activity. For instance, if an algorithm notices an odd trend of high-value transactions coming from an account that has normally only had low-value transactions in the past, it may flag the transaction as possibly fraudulent.
Another example is the use of natural language processing (NLP), which may be used to analyze customer complaints or conversations in order to find suspicious terms or patterns that may point to probable fraud activities.
AI can help with credit risk assessment in addition to fraud detection. Systems with artificial intelligence (AI) can assess a variety of information about people and companies, including financial and non-financial data, to forecast their chance of defaulting on loans.
AI is a useful tool for risk assessment and fraud detection in fintech because of its capacity to evaluate enormous volumes of data rapidly and reliably. The use of AI for risk assessment and fraud detection is currently prevalent among fintech organizations, and it is anticipated to grow further in the future.
Examples of companies using AI for risk assessment and fraud detection:
Capital One: Capital One uses AI-powered systems for fraud detection, analyzing large amounts of data to identify patterns and anomalies that may indicate fraudulent activity.
Experian: Experian is using AI to assess credit risk and detect fraudulent activity. The company’s AI-powered systems can analyze financial and non-financial data to predict an individual or business's likelihood of defaulting on a loan.
Jumio: Jumio is a fintech company that uses AI-powered identity verification to prevent fraud. Its platform can analyze ID documents, facial biometrics, and other forms of identification to ensure that a person is who they claim to be.
Examples of companies using AI for Investment management:
Robinhood: Robinhood, a zero-fee brokerage firm is using AI to power its ‘robo-advisory’ service. This service uses machine learning algorithms to analyze data and make recommendations for investment portfolios based on an individual's risk tolerance and investment goals.
Wealthfront: Wealthfront is an online financial management company that uses AI to provide personalized investment advice. The company's AI-powered platform can analyze a wide range of data to create customized investment portfolios for each client.
SigFig: SigFig is a fintech company that offers a robo-advisory platform for investment management. Its AI-powered platform can analyze data on individual stocks and provide investment recommendations based on the risk appetite and investment goals of the client.
AI and Portfolio Management:
Investment management, especially in the fields of portfolio management and stock trading, is one of the key areas where AI is being employed in fintech.
By evaluating a massive quantity of data on specific stocks, bonds, and other assets to evaluate their projected return and risk, AI-powered systems can help with portfolio management. Based on each client's unique risk tolerance and investment objectives, this information may be utilized to develop personalized investment portfolios for them.
Additionally, by utilizing machine learning algorithms for stock trading, AI may be applied. These algorithms are able to examine stock-specific data and forecast future price alterations. Buying and selling choices may be made using this information, potentially improving returns on investment.
The emergence of Robo-Advisors, online platforms that employ algorithms to deliver automated, algorithm-driven financial planning services with little to no human oversight, is one of the most noteworthy and prevalent examples of AI's use in the fintech sector.
Examples of Robo-Advisers that use AI
Wealthfront: Wealthfront uses AI-powered algorithms to analyze data and create customized investment portfolios for each client based on their individual risk appetite and investment goals.
SigFig: SigFig uses AI-powered algorithms to provide personalized investment advice to its clients. The platform can analyze data on individual stocks and provide investment recommendations based on the client's risk appetite and investment goals.
Betterment: Betterment uses AI-powered algorithms to provide investment advice and management services to its clients. The platform can analyze data on individual stocks and provide recommendations for a diversified investment portfolio based on the client's risk appetite and investment goals.
AI-powered systems are growing more complex and are now able to evaluate enormous volumes of data and forecast future price changes. It is anticipated that as these systems develop, they will become increasingly more useful tools for stock trading and portfolio management.
AI and Customer Service:
The customization and customer service aspects of finance are another area where AI is being applied. Natural language processing (NLP) and machine learning techniques can be used by AI-powered systems to comprehend and respond to client enquiries sent through channels like chatbots or virtual assistants.
As AI-powered systems are capable of handling several client questions concurrently and providing immediate replies, this may significantly increase the speed and efficiency of customer support. By examining data on each client's preferences and transactions to generate customized recommendations and offers, AI may also be used to personalize consumer experiences.
Examples of companies using AI for customer service and personalization in fintech include:
American Express: American Express uses AI-powered chatbots to assist customers with account management and answer frequently asked questions.
Wells Fargo: Wells Fargo uses AI-powered virtual assistants to provide customer service through its mobile app.
USAA: USAA uses AI-powered chatbots to assist customers with account management and answer frequently asked questions
AI may be utilized for personalisation in addition to chatbots by employing recommender systems. To offer individualized suggestions for goods and services, these systems can evaluate data on a customer's previous purchases and interests.
By offering quick and accurate answers to questions and individualized recommendations, the use of AI in customer service and customization has the potential to significantly improve the customer experience. More fintech organizations are anticipated to begin use AI in similar ways to enhance their operations and better serve their consumers as technology develops and AI gets more advanced.
Explanation of how AI can improve customer service through natural language processing and chatbots
Using chatbots and natural language processing (NLP) in finance is one way AI may enhance customer support. Chatbots are computer programs that can imitate conversations with human users through text or voice interactions. NLP is a subset of AI that enables robots to comprehend and interpret human language.
NLP and chatbots can work together to provide a potent customer care solution for the finance industry. NLP enables the chatbot to comprehend consumer enquiries and reply to them in a manner like to that of a human customer support person. By responding to queries quickly and accurately without requiring a human person to be on hand all the time, this enhances the customer experience.
A website, a mobile app, social media, and messaging services like WhatsApp and Facebook Messenger are just a few of the venues where chatbots may be embedded. This makes it simpler for clients to get customer support when they are on the road and allows them to use the channel of their choice.
Additionally, a variety of consumer requests, such as account management, balance inquiries, and frequently asked questions, may be handled by chatbots. This can assist to increase the overall effectiveness of the customer service process by freeing up human customer service personnel to address more complicated situations.
The use of NLP and chatbots in customer service can greatly improve the customer experience by providing fast and accurate responses to inquiries. As technology continues to advance and NLP becomes more sophisticated, it's expected that even more fintech companies will start using AI in similar ways to improve their operations and better serve their customers.
Examples of businesses adopting AI for personalisation and customer service in fintech include:
American Express: American Express utilizes chatbots that are driven by AI to help consumers manage their accounts and provide answers to commonly asked inquiries. Customers can easily obtain the assistance they want on their preferred platform by using the chatbot, which is accessible through the American Express website, mobile app, and social media channels.
Wells Fargo: Using its mobile app, Wells Fargo offers client care through virtual assistants that are powered by AI. Customers may view account details, check balances, and carry out activities including money transfers between accounts via the virtual assistant.
USAA: USAA utilizes chatbots that are powered by AI to help consumers manage their accounts and provide answers to commonly asked inquiries.
To give consumers prompt and accurate replies, the chatbot may answer questions about account balances, transactions, and other account-related matters.
BBVA: Through its mobile app, internet, and social media platforms, BBVA offers client care using virtual assistants that are driven by AI.
Customers may utilize the virtual assistant to check account balances, view account information, and complete operations like bill payments or money transfers across accounts.
N26: N26 bank has created a virtual personal financial assistant named Max using artificial intelligence (AI). Max may assist you with your banking requirements, such as automating saves, locating the best offers, or providing individualized recommendations.
These are just a handful of the numerous businesses that use AI to personalize and enhance customer service in the financial industry. More fintech organizations are anticipated to begin use AI in similar ways to enhance their operations and better serve their consumers as technology develops and AI gets more advanced.
AI and Credit Underwriting:
The usage of AI in the fintech industry also extends to credit underwriting. The practice of assessing a borrower's or a business's creditworthiness is known as credit underwriting.
Systems driven by artificial intelligence (AI) can help with this process by evaluating enormous quantities of data, including financial and non-financial data, to forecast the possibility of a person or company defaulting on a loan. Data including income, credit history, work history, and social media usage may be included.
As AI-powered systems can analyze massive volumes of data fast and make judgements on loan applications more precisely than conventional techniques, its use in credit underwriting can significantly increase the speed and efficiency of the process. Additionally, since AI-powered systems base their conclusions on data rather than human judgment, they can assist in lowering the possibility of human bias in the loan application process.
The following are some examples of fintech businesses employing AI for credit underwriting:
LenddoEFL: LenddoEFL utilizes AI to assess a person's creditworthiness who may not have a typical credit history and who lives in a developing country.
An individual's chance of defaulting on a loan may be predicted using the company's AI-powered technology by analyzing data from social media and mobile phone usage.
ZestFinance: ZestFinance using AI to assess a person's creditworthiness even if they do not have a traditional credit history.
A person's chance of defaulting on a loan may be predicted using data from a variety of sources by the company's AI-powered system.
Kabbage: Kabbage employs artificial intelligence to assess small enterprises' creditworthiness. A business's chance of defaulting on a loan may be predicted using data from social media, accounting software, and other sources by the company's AI-powered system.
Using AI to credit underwriting has the potential to significantly increase the speed and effectiveness of the loan application process while lowering the likelihood of human bias. It's anticipated that even more fintech organizations will begin employing AI in credit underwriting as technology develops and AI gets more advanced in order to enhance operations and better serve their clients.
Explanation of how AI can assist with evaluating creditworthiness
By analyzing vast quantities of data to forecast the possibility of a person or company defaulting on a loan, AI may help in evaluating creditworthiness. "Credit scoring" refers to this method.
Machine learning algorithms are one means through which AI may help with credit scoring. These algorithms may examine information about a person or a company, such as income, credit history, work history, and social media activity, to forecast the risk that they would not repay a loan.
AI may also be used to find patterns and abnormalities in data, which helps in detecting elements that can point to a greater default risk.
For instance, if a person has a history of making late payments on their credit card bills, an AI-powered system may identify them as having a higher risk of default.
The use of natural language processing (NLP) to evaluate unstructured data, such as a customer's social media profiles, blog posts, or emails, to get a better understanding of the customer's financial behavior and creditworthiness is another use of AI in the credit underwriting process.
The danger of human bias throughout the loan application process can be decreased with the use of AI in credit underwriting since these systems base their choices on data rather than human judgment.
This promotes the fairness and objectivity of lending choices.
All things considered, AI can help with creditworthiness evaluation by analyzing massive volumes of data, spotting patterns and abnormalities in the data, and forecasting the possibility of default.
By doing so, the danger of human bias is diminished and the efficiency and accuracy of the credit underwriting process are considerably improved.
Examples of companies using AI for credit underwriting in fintech include:
LenddoEFL: LenddoEFL employs artificial intelligence to assess a person's creditworthiness in a developing nation who might not have a traditional credit history.
An individual's chance of defaulting on a loan may be predicted using data from social media and mobile phone behavior using the company's AI-powered approach.
ZestFinance: ZestFinance assesses the creditworthiness of those who might not have a typical credit history using artificial intelligence.
The business' AI-powered system may examine information from a variety of sources to forecast a person's chance of missing a loan payment.
Kabbage: Kabbage employs artificial intelligence to assess small enterprises' creditworthiness.
A business's chance of defaulting on a loan may be predicted using data from social media, accounting software, and other sources by the company's AI-powered system.
LendUp: LendUp use AI to assess a person's creditworthiness even if they don't have a traditional credit history.
Their AI-powered system can assess information on an individual's past employment history, income, and other criteria to forecast whether they would fail on a loan.
OnDeck: OnDeck employs artificial intelligence (AI) in their underwriting process to assess small businesses' creditworthiness, allowing them to extend loans to individuals that were previously deemed too risky by the traditional banking sector.
These are just a handful of the numerous businesses in the fintech sector that use AI for credit underwriting.
More fintech businesses are anticipated as technology develops and AI gets more advanced.
Challenges and limitations of AI in fintech
Although AI has the potential to transform the financial sector, its use is not without difficulties and restrictions.
The requirement for vast quantities of high-quality data to develop and evaluate AI models is one of the major obstacles.
The availability of data in the financial sector may be constrained by worries about data privacy and security, making it challenging to create AI systems that work well.
The possibility for prejudice in AI systems is another problem.
An AI model may produce biased choices if the data it was trained on is skewed.
For instance, if the historical data used to train the AI model contains biased information about particular groups of individuals, the machine may mimic prior discriminatory lending practices.
Another drawback is the interpretability of the models; it is challenging to comprehend and articulate how a sophisticated AI model comes to a certain conclusion.
This lack of interpretability complicates regulatory compliance and can make it harder for businesses to completely comprehend and believe in the judgments made by their AI systems.
The fact that some businesses, particularly financial services, are highly regulated can also make it difficult to incorporate new technology since it is necessary to assure compliance with rules and laws.
Finally, it is challenging to fully automate many financial activities due to the complexity of financial goods, transactions, and laws. As a result, financial organizations may need to retain human expertise to handle complicated circumstances.
Data privacy and security concerns
When it comes to implementing AI in fintech, data security and privacy are major considerations.To keep clients' trust and adhere to rules, the financial sector must secure sensitive information it manages, such as individual financial information and private transaction details.
One significant worry is the potential for data breaches, in which unauthorized people access private data. These intrusions may be the result of hacking, phishing schemes, or other nefarious actions. Strong security measures must be in place for businesses to secure the data they gather and hold in order to fight this.
Another issue is the potential for data leakage, which occurs when private data is unintentionally or maliciously disclosed to uninvited parties.
This might happen due to inadequate security measures or human mistake, such as an employee sharing information with the incorrect person.
In addition, several nations have special data privacy laws, like the GDPR, that businesses must follow in order to safeguard consumers' personal information.
It can be difficult to do this since AI models frequently need access to vast volumes of data, which must be gathered, processed, and kept in accordance with these laws.
Difficulty in explaining the decision-making processes of AI
The difficulty of articulating the decision-making processes of AI models is one of the major obstacles to the use of AI in finance. Many AI models are referred to as "black boxes" because it is challenging to comprehend how they make decisions, particularly those that employ sophisticated machine learning methods. This lack of interpretability might make it difficult for businesses to comprehend and believe the judgments made by their AI systems. It can also make it difficult to comply with regulations.
Regulators in the financial sector may demand that businesses be able to describe the decision-making processes of their AI systems, particularly if those judgments have a substantial impact on clients. For instance, a loan application approval or denial decision made by an AI-powered lending system must be explicable. Regulation compliance may not be possible if the system is viewed as a "black box" and its decision-making process cannot be explained.
There are several ways to address this problem, some of which include:
Model interpretability: Improving the interpretability of AI models to make their decision-making processes easier for humans to comprehend.
Explainable AI: Explainable AI is a research-in-progress technique that tries to develop AI systems that can describe their decision-making process in ways that are intelligible to humans.
Regulator-facing AI systems:Development of AI systems that can explain their decision-making process to authorities and are geared toward compliance with regulatory regulations.
The deployment of AI in fintech faces considerable challenges, and businesses must take action to overcome these challenges if they are to preserve public confidence in their AI systems and adhere to legal requirements.
Need for more advanced AI techniques to handle complex financial transactions
The requirement for more sophisticated AI methods to manage complicated financial transactions is another difficulty in the application of AI in fintech. Due to the complexity of financial goods and services, typical AI approaches might not be adequate to fully automate all financial procedures.
For instance, it might be difficult for AI models to completely comprehend and make predictions about the underlying assets and market circumstances in the case of financial derivatives, which are intricate financial contracts used to control risk.
Similarly, it's difficult for AI to completely comprehend the underlying assets and to estimate the credit risk for Credit Default Swaps (CDS), which are financial contracts used to transfer the credit risk of a third party.
The current state of AI technology prevents many other financial transactions from being totally automated because they are too complicated.
This comprises:
Pricing of sophisticated financial instruments like options and swaps
Stress testing and risk management for sophisticated financial products
Gaining insights into industry patterns through the analysis of large data and unstructured data, including news articles and social media posts
Companies may need to use more sophisticated AI methods, such reinforcement learning, or employ a combination of AI and human specialists to manage these complicated financial transactions. To create the requisite AI capabilities, this could necessitate more considerable investments in research and development.
Comments